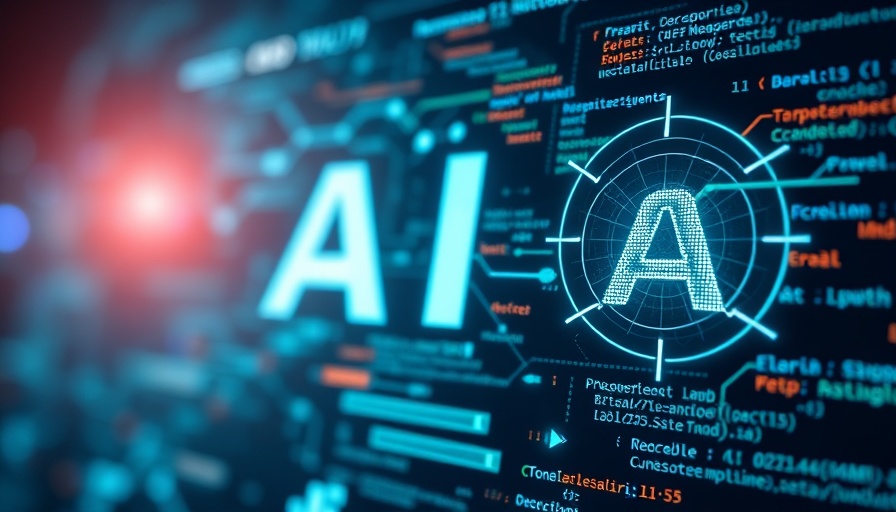
Revolutionizing AI Training: The Indoor Training Effect
Artificial intelligence (AI) has markedly transformed sectors from healthcare to entertainment, all while consistently pushing the boundaries of its capabilities. A groundbreaking study led by researchers from the Massachusetts Institute of Technology (MIT) reveals a fascinating insight into AI training—the 'indoor training effect.' This emerging concept suggests that training AI agents in environments dissimilar to where they are eventually deployed can yield superior results than previously believed.
Understanding the Indoor Training Effect
In conventional wisdom, AI agents are trained in environments that closely mimic their deployment scenarios. This alignment aimed at minimizing discrepancies reduces uncertainty or 'noise' to improve learning outcomes. However, the research dispels this age-old belief, indicating that agents trained in less complex, more controlled environments often exhibit enhanced performance in chaotic settings. The team observed this effect during experiments involving Atari games, where they modified game conditions to increase unpredictability.
Real-World Applications and Implications
The implications of the indoor training effect could be profound, especially in sectors where performance under uncertainty is critical, such as autonomous vehicles, robotics, and healthcare systems. For instance, robots designed to assist in household chores—like scrubbing a sink or taking out trash—could potentially be trained in a factory setting, where interactions and obstacles are far more manageable. Subsequently, when deployed at a real home, these agents might adapt better to unforeseen circumstances thanks to their diverse, carefully structured training.
Parallel Examples: Lessons From Sports
To illustrate this phenomenon further, consider how athletes train. Tennis players often practice indoors where variables such as wind, weather changes, and court surfaces are controlled. An indoor athlete may build a repertoire of strokes with precision, only to encounter real-world challenges outside. Similarly, AI agents experiencing controlled simulations may develop a foundational skill set that allows them to adjust more readily when faced with unpredictable, real-world variables.
The Future of AI Training: A New Paradigm?
The research advocates for rethinking our approach to AI training protocol. Moving forward, engineers and researchers may benefit from designing training programs that leverage environments distinct from typical operating conditions. As co-author Spandan Madan articulated, "This is an entirely new axis to think about," which prompts us to reevaluate our testing criteria moving forward. Developing AI agents in carefully curated environments can expand versatility and adaptability, ultimately leading to robust performance in dynamic conditions.
Challenges and Considerations Within AI Training
Of course, implementing this paradigm shift comes with its own set of challenges. Training times may increase, and there may be higher costs associated with creating accurate indoor training models. Furthermore, determining the most effective divergence from real-world environments could pose complex questions that demand a tailored approach to each unique AI application.
Actionable Insights for AI Developers
For developers and engineers in the AI field, this research underscores the need for flexibility and creativity in training methodologies. Rather than adhering strictly to familiar training methods, embracing the potential of diverse environments may yield innovative solutions. Exploring indoor environments where uncertainty is minimized could become a best practice, promoting not just efficiency but also improved AI capabilities.
Conclusion: Embracing Change in AI Training Methodologies
The indoor training effect is more than a mere academic observation; it represents a transformative shift in how we think about training AI agents. As further research unfolds, staying informed on these developments can equip image professionals, developers, and researchers with the tools to enhance AI's adaptability. Embracing this paradigm shift will allow for smarter AI that can navigate the complexities of real-world scenarios, ultimately bringing us closer to achieving our pioneering aspirations in artificial intelligence.
Write A Comment