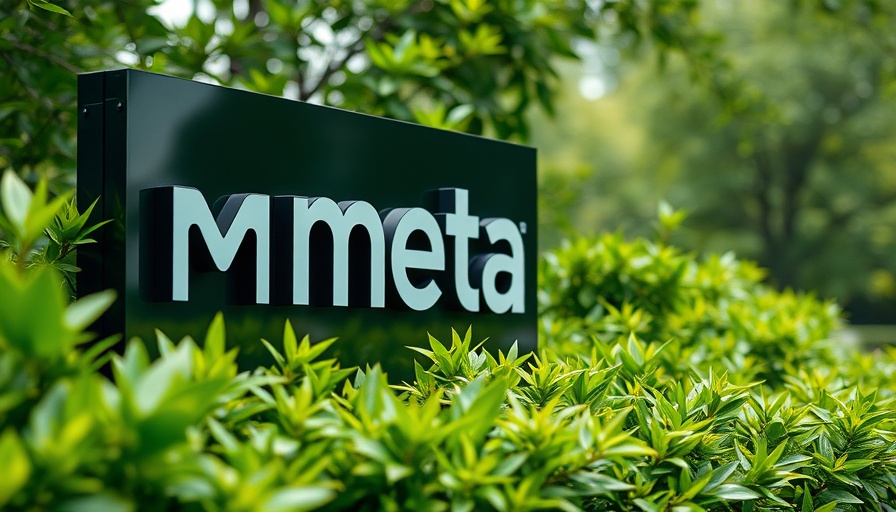
Meta's New AI Model Maverick: A Closer Look
Meta has recently released its flagship AI model, Maverick, generating considerable attention in the artificial intelligence community. Ranking second on the LM Arena test, Maverick's performance has sparked discussion regarding its benchmarking practices. While it may appear successful at first glance, the underlying details reveal a more complex picture.
Understanding LM Arena and Its Limitations
LM Arena is designed to assess AI model outputs through human comparisons to determine preferences. However, this method has drawn criticism over its reliability. Researchers have previously pointed out that results from LM Arena don't always align with real-world applications. Meta's approach to tailoring the Maverick model for this benchmark seems to raise questions about the authenticity of its operational performance.
What Distinguishes the Versions of Maverick?
One of the most significant concerns is the difference between the version of Maverick available on LM Arena and the one accessible for developers. Meta's announcement highlighted that the LM Arena version is an "experimental chat version" optimized for conversational contexts. In contrast, the standard version that developers utilize does not carry these enhancements. This customization could lead developers to misinterpret the true capabilities of Maverick when applying it in varied scenarios.
The Implications for Developers and Users
For developers eager to deploy Maverick in projects, understanding the disparity between the two versions is crucial. It impacts how they might predict how the model performs across different contexts. If a customized benchmark leads to overly optimistic expectations, developers may risk encountering unexpected challenges once they implement the model in real-world situations.
Social Media Reaction: A Closer Look at User Observations
Feedback from AI researchers on social media platforms such as X (formerly Twitter) has underlined the distinctions between the versions. Users noted that the LM Arena model appears to be more emoji-heavy and less concise than the downloadable variant. This disparity could affect its usability, as developers and end-users might prefer different features in varying contexts.
Benchmarking: The Necessity for Transparency
In the tech landscape, transparent benchmarking is vital for credibility. Companies should aim to unveil how models are assessed and the criteria that dictate their performance outcomes. Tailoring models specifically for benchmark tests can mislead consumers about their capabilities and risks eroding trust in AI products. For more reliable evaluations, eying multiple evaluation sources rather than focusing solely on one could provide a holistic view.
Future Insights: What Lies Ahead for AI Benchmarking?
Given the existing challenges surrounding AI benchmarks like LM Arena, future trends may lead to the development of more standardized assessment methods. As AI technologies evolve, establishing widely accepted metrics and evaluation standards could enhance trust and understanding among developers and users alike.
Calls for Responsible AI Development
As companies step into this progress, they must remain vigilant about responsible AI development. This involves addressing ethical concerns related to AI transparency and ensuring users can rely on showcased performance metrics. Creators of AI tools must be aware that benchmarks shape perceptions, and ensuring accuracy can lead to advances within the sector.
Conclusion: Staying Informed in a Rapidly Evolving AI Landscape
In an era where AI continues to transform industries, it is crucial for stakeholders to stay informed about the nuances within benchmarks and the models they utilize. By fostering understanding and engagement within the AI community, we pave the way for more reliable technology that meets the actual needs of users.
Write A Comment