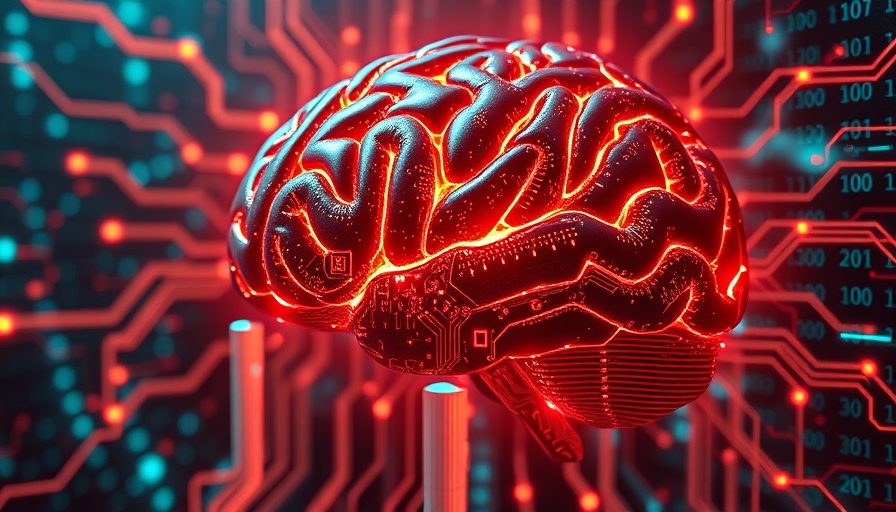
Understanding Large Language Models: A Leap Towards Human-Like Reasoning
In recent years, large language models (LLMs) have transcended the limitations of earlier iterations that could only process text. These models now undertake a multitude of diverse tasks that include understanding various languages, generating computer code, solving mathematical problems, and even interpreting audio and visual inputs. Remarkably, emerging research from the Massachusetts Institute of Technology (MIT) reveals that LLMs integrate diverse types of data similarly to human cognition. By employing a central processing mechanism akin to the human brain’s semantic hub, these models demonstrate a sophisticated ability to reason about disparate data types.
The Semantic Hub: A Brain-Like Processing Mechanism
Human thought processes are often funneled through a semantic hub located in the brain's anterior temporal lobe. This hub integrates semantic information from various modalities—visual, tactile, and auditory—via modality-specific spokelike connections. MIT researchers have found that LLMs employ a comparable processing style. For example, an LLM may process English as its primary language while simultaneously reasoning about inputs in Japanese or analyzing coding commands. This method not only showcases the model’s versatility but also suggests that LLMs can innovatively combine knowledge from multiple languages and formats, facilitating richer interpretations and outputs.
Expanding Our Understanding of LLM Functions
As the authors of this cutting-edge study probe deeper into LLM functionalities, they aim to demystify what is often referred to as the 'black box' of LLM operations. As Zhaofeng Wu, the lead author of the research, notes, although LLMs can achieve impressive performance metrics, our grasp of their internal mechanisms remains limited. Enhancing our understanding of these processes could eventually yield LLMs that are better adapted for handling a wide array of data types with increased efficiency.
Interventions in the Semantic Hub: A Busy Lesson in Control
The study also illuminated potential strategies for intervention within the model’s semantic hub. Through linguistic manipulation using the model's dominant language, researchers demonstrated that they could influence the model’s outputs even when it processes data in alternative languages. This highlights a promising avenue for scientists looking to refine LLM behaviors, potentially augmenting how these models draw on diverse information.
Integrating Knowledge Across Modalities: Commonalities and Complexities
Building on prior studies that hinted at English-centric LLMs predominantly utilizing English knowledge, Wu and colleagues expanded their focus to explore how these models can process varied data efficiently without duplicating existing knowledge across languages. They discovered that the initial phases of the LLM process data in relation to its dominant language, before transitioning into a broader, more abstract representation of information, treating diverse stimuli—text, images, and audio—equally.
Future of Multimodal LLMs and Real-World Applications
Insights drawn from this research have substantial implications for the future of multimodal LLMs. By refining the semantic hub interaction, researchers aim to boost the models' efficiency without compromising their accuracy across languages. The potential application of these insights could transform fields requiring intricate data interpretation, such as multilingual customer service, automated content generation, and even complex scientific research.
The Challenge of Cultural Context: What’s Lost in Translation?
While the ability to process diverse data types is a significant advantage, the study raises an intriguing question about cultural relevance and knowledge specificity. As Wu suggests, not all concepts may be easily transferable across languages and modalities. There is an urgent need to consider how to efficiently process information while respecting cultural nuances that may not translate well. Future research may need to navigate these subtleties to create LLMs that both share knowledge efficiently and account for unique cultural contexts.
Final Thoughts: Boosting Efficiency by Understanding Cognitive Models
The pursuit of better comprehension of LLMs and their operational mechanisms could unlock new layers of efficiency and adaptability. As we venture into an era dominated by generative AI, being equipped with the necessary knowledge of these models will be crucial for researchers and practitioners alike. Ultimately, the question remains: how can we balance efficiency with the richness of localized knowledge and cultural competence?
Write A Comment