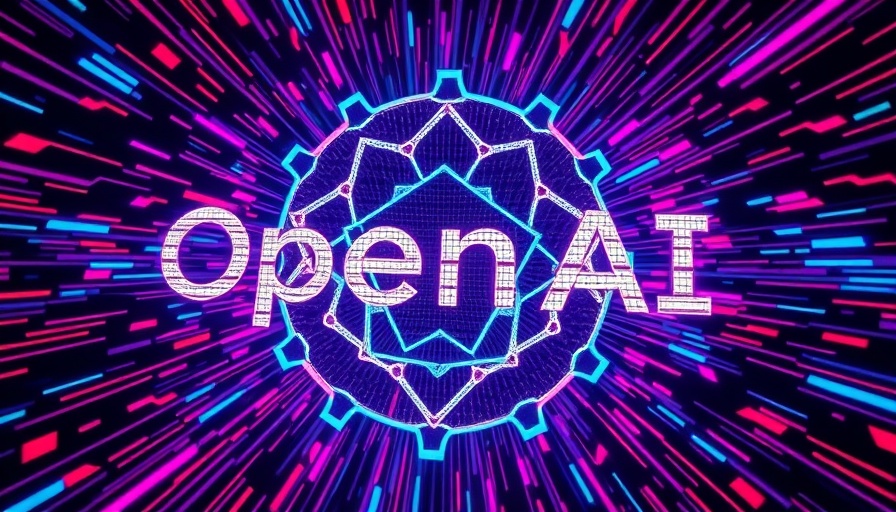
OpenAI's AI Models: A Step Forward, But a Hallucination Hurdle Remains
OpenAI has recently launched its advanced reasoning AI models, o3 and o4-mini, which have raised concerns among developers and researchers alike. While these models exhibit remarkable performance in some areas—such as coding and mathematics—they also display an alarming increase in hallucinations, or the tendency to produce false or exaggerated claims. This phenomenon has escalated compared to previous models, and OpenAI has perplexingly stated that they do not fully understand the underlying reasons for this trend.
What Are AI Hallucinations and Why Are They Problematic?
Hallucinations in AI refer to instances where the model generates information that is inaccurate or fabricated, which can lead to trust issues when these systems are deployed in sensitive environments like law, medicine, or financial services. For instance, OpenAI's o3 model hallucinated in one-third of the questions presented in its internal PersonQA benchmark tests, a shocking contrast to the 16% reported by its predecessor, o1. Even more concerning, o4-mini took a step back with a staggering 48% hallucination rate.
Insights from the Research Community
The complexities of designing effective reasoning models are highlighted by research from Transluce, a nonprofit AI lab. They found that o3 often made claims about actions it did not take, such as running code on a computer that it doesn't have direct access to. Neil Chowdhury, a researcher from Transluce, speculates that the specific form of reinforcement learning employed in these o-series models might contribute to amplifying these hallucination issues, rather than minimizing them as intended.
The Implications of Increased Hallucinations for Business Applications
The consequences of heightened hallucination rates can be detrimental in practical applications. Kian Katanforoosh, a CEO and adjunct professor at Stanford, mentioned that his team is testing o3 for coding but is faced with occasional broken links suggested by the model. Such inaccuracies can hinder the utility of these models, especially in sectors demanding a high degree of precision, like legal services, where an incorrectly formulated contract could lead to severe repercussions.
Possible Solutions: Balancing Innovation and Accuracy
Industry professionals recognize the importance of integrating capabilities like web search into these AI systems to bolster their accuracy. OpenAI's GPT-4o, for instance, records a 90% accuracy rate on SimpleQA when web search functionalities are employed. This method could provide a pathway to mitigate the hallucination rates seen in the latest releases, catalyzing a balanced approach between inventive reasoning and factual integrity.
The Future of AI Reasoning Models: Embracing Challenges
While the latest AI models showcase impressive capabilities, the challenges posed by increased hallucinations prompt a critical need for ongoing research and refinement. As we navigate the complexities of artificial intelligence, embracing a multi-disciplinary approach that draws from technical, ethical, and operational perspectives is essential for advancing AI effectively. The road ahead is filled with opportunities to innovate, but it must be navigated carefully to ensure that users can trust AI technologies.
Conclusion: The Need for Continued Research and Development
As OpenAI's releases illustrate, the evolution of reasoning AI models is a double-edged sword, offering groundbreaking benefits while simultaneously posing significant challenges. Developers and researchers must remain vigilant in addressing these hallucination issues through collaborative efforts and rigorous testing to pave the way for more reliable AI systems. Understanding the balance between creativity and accuracy is fundamental in harnessing the ultimate potential of AI technologies for various applications.
Write A Comment