Did you know that 90% of the world’s data was generated over the past two years? As we barrel into the future, understanding the technological giants—Machine Learning v Deep Learning—becomes crucial. Dive into the comprehensive exploration of these transformative technologies and uncover their groundbreaking potentials.
Unlocking the Potential: Understanding Machine Learning v Deep Learning
The terms Machine Learning and Deep Learning often spring up in discussions about artificial intelligence, but they signify distinct processes. Machine Learning lies at the heart of AI, allowing systems to learn from structured data while deep learning takes this process further by mimicking the neural processes of the human brain to analyze unstructured data. Together, they create a sophisticated synergy that powers today's AI-driven innovations.
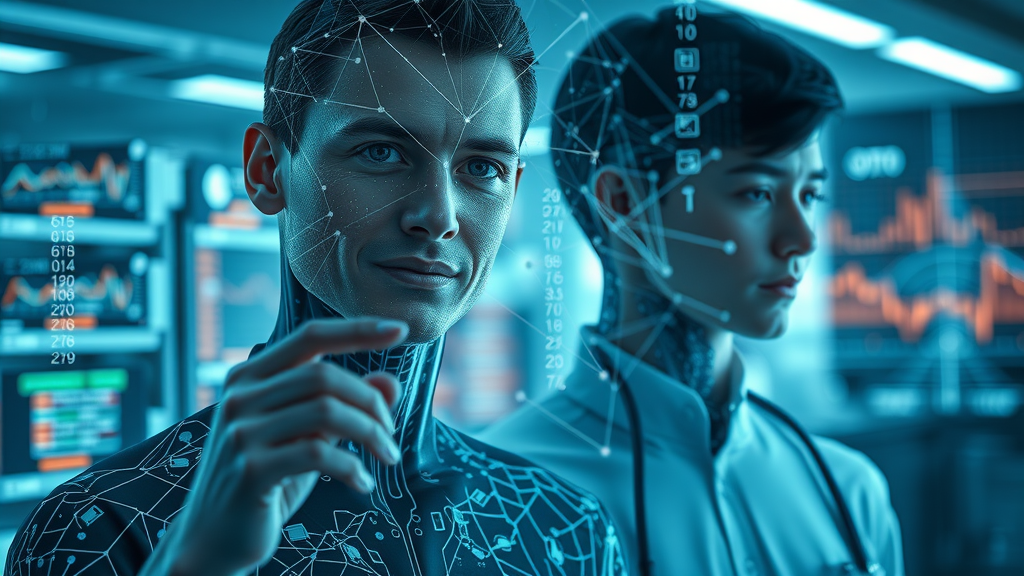
The Power of Statistics: A Closer Look at Machine Learning v Deep Learning
Machine Learning forms the bedrock of artificial intelligence by employing algorithms to parse data, learn from it, and make informed decisions. Conversely, Deep Learning utilizes neural networks with multiple layers, often requiring more training data but offering higher accuracy for tasks like image and speech recognition. The distinction lies in their processing capabilities and the complexity of tasks they can handle, highlighting the continuous evolution of AI.
Deep Learning: The Revolution of Artificial Neural Networks
Deep Learning stands as a revolutionary leap in AI, largely due to its use of artificial neural networks. These models are designed to imitate the workings of the human brain, composed of interconnected nodes, much akin to neurons. This intricate network structure allows systems to solve complex patterns in data and make predictions beyond what's achievable by traditional machine learning.
Exploring Deep Learning and its Core Mechanics
The marvel of Deep Learning lies in its ability to process vast volumes of data through numerous hidden layers, known as the hidden layer, within its neural networks. These layers extract features automatically from raw data, significantly reducing the need for manual human intervention. Deep Learning algorithms thrive in tasks where intricate data interrelationships are vital, such as natural language processing and self-driving technologies.
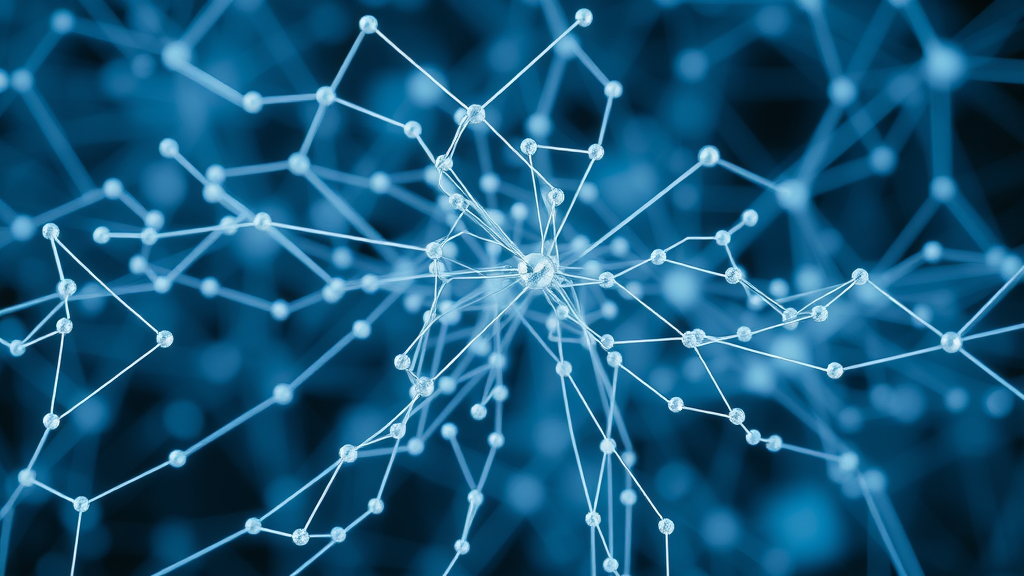
The Role of Neural Networks in Deep Learning
The foundation of deep learning rests on the elaborate network of neural networks. These artificial neural networks operate analogously to the human brain, allowing systems to learn from data independently. The networks' vast connectivity and depth enable them to perform complex operations, such as recognizing patterns in images, understanding natural language, and performing predictive analytics.
Applications of Deep Learning in Today's World
In our rapidly evolving tech landscape, deep learning is a pivotal force behind many advancements. From powering voice-activated assistants to enabling facial recognition software, the applications are vast and varied. It also significantly enhances predictive models in healthcare, finance, and beyond, illustrating its versatility and potential in reshaping industries.
Machine Learning: The Foundation of Artificial Intelligence
At the core of artificial intelligence, Machine Learning offers a foundation upon which AI systems are built. It is the precursor to deep learning, providing the necessary frameworks and algorithms that improve tasks based on previous data interactions.
Fundamentals of Machine Learning
Machine Learning relies on algorithms that identify patterns within data. These machine learning models learn from training data, improving their decision-making abilities without explicit programming. As they ingest data, these models become more effective over time, offering a dynamic and responsive AI experience.
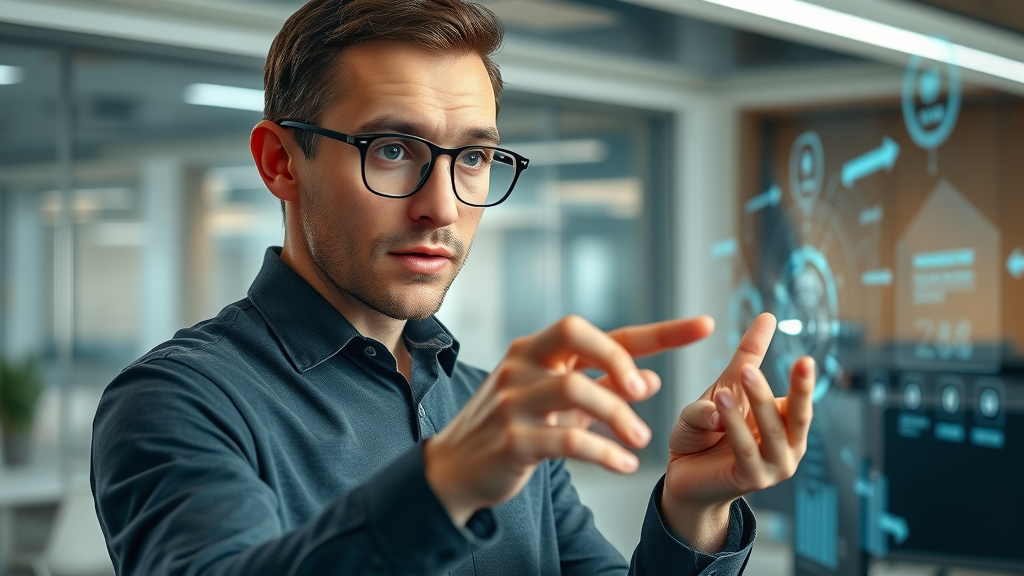
How Neural Networks Fuel Machine Learning
The integration of neural networks within machine learning frameworks has catalyzed a significant leap forward in AI development. These networks serve as a bridge, allowing the processing of more complex datasets and enhancing the precision and intelligence of machine learning systems.
Real-World Applications of Machine Learning
Machine Learning plays an indispensable role in today's world, driving improvements in fields like recommendation systems, fraud detection, and predictive maintenance. Its capability to learn from structured data and adapt accordingly makes it a cornerstone of innovations across various domains, continuously pushing the boundaries of what's possible.
Comparing Machine Learning and Deep Learning
Pitting machine learning and deep learning against each other reveals nuanced differences that shape their applications and capabilities. While both are integral to AI, the complexity, data requirement, and processing power distinguish them significantly.
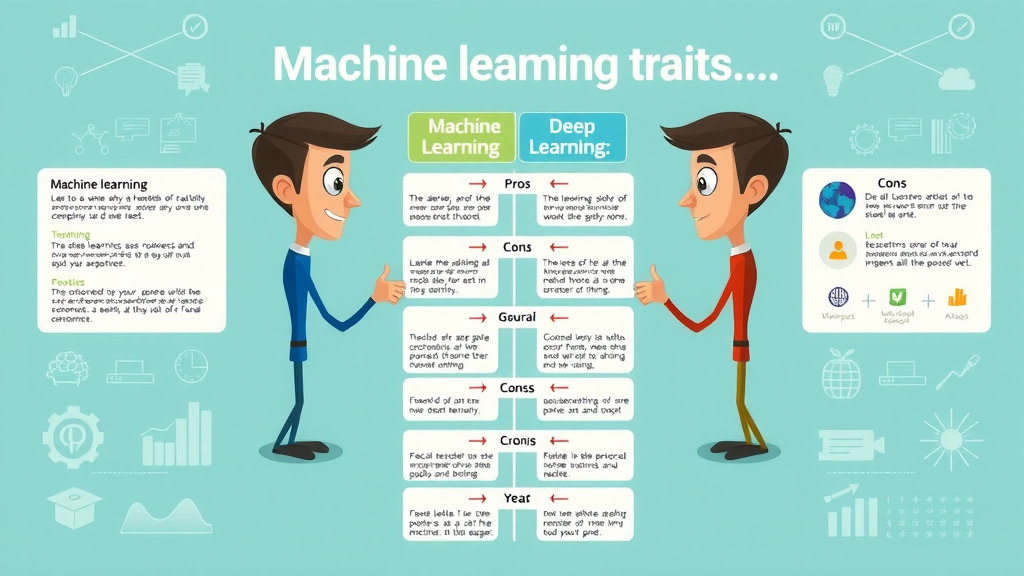
Key Differences Between Machine Learning and Deep Learning
The core difference between machine learning and deep learning lies in their approach to data processing. Machine learning relies on algorithms trained on structured data, while deep learning delves into unstructured data through its intricate neural networks. While machine learning models require human intervention for feature extraction, deep learning networks autonomously discern features through their hidden layers.
Benefits and Limitations of Each Approach
A critical analysis of machine learning v deep learning reveals their strengths and limitations. Machine learning offers quicker setup times and less computational power but may lack the insight derived from vast datasets. Conversely, deep learning excels in handling large data volumes, providing superior accuracy, but often demands greater computational resources and longer training times.
Understanding Learning Models in AI
In AI, learning models form the backbone of intelligent systems. These models determine how data is processed and insights are gleaned. With both machine learning algorithms and deep learning algorithms, systems can tailor operations, improve efficiencies, and drive forward-thinking solutions across industries.
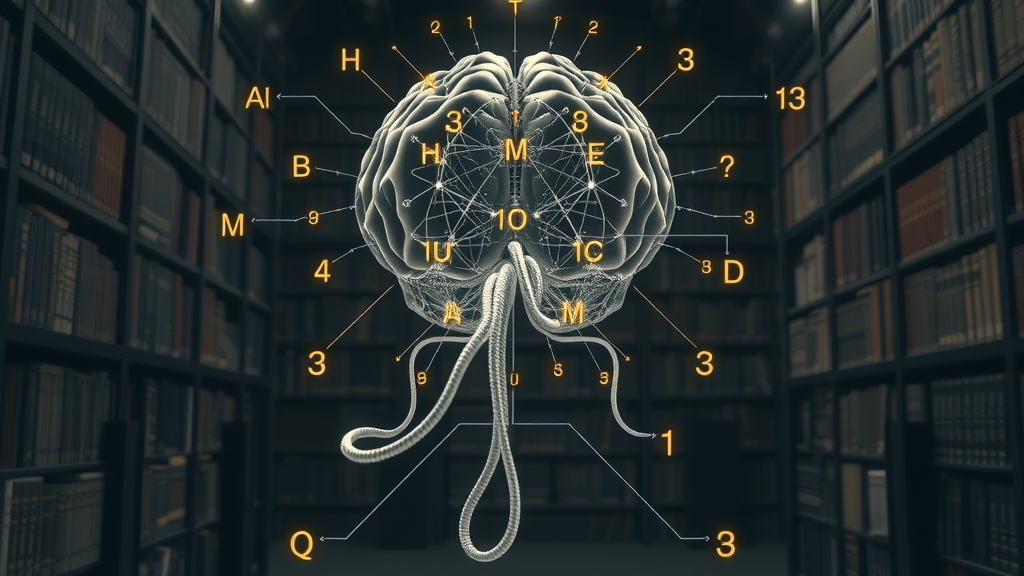
Technological Advancements Driven by Machine Learning and Deep Learning
Machine learning and deep learning have propelled numerous advancements in the tech world, significantly impacting AI research and development. These technologies harness the power of data to foster innovative solutions and push the boundaries of what’s possible within the realm of technology.
The Impact of Machine Learning on AI Research
Machine learning has influenced AI research by providing robust methods to analyze and predict complex data patterns. It has driven advancements in adaptive learning techniques, enhancing automation, and enabling intuitive human-computer interactions, creating a ripple effect across research avenues.
Deep Learning's Role in Advancing AI Technologies
Deep Learning paves the path for cutting-edge AI technologies, cementing its role in developing language translators, robotic systems, and diagnostic tools. Its capability to process and analyze vast quantities of unstructured data efficiently facilitates breakthroughs across various technological fronts.
People Also Ask
What is the difference between deep learning and machine learning?
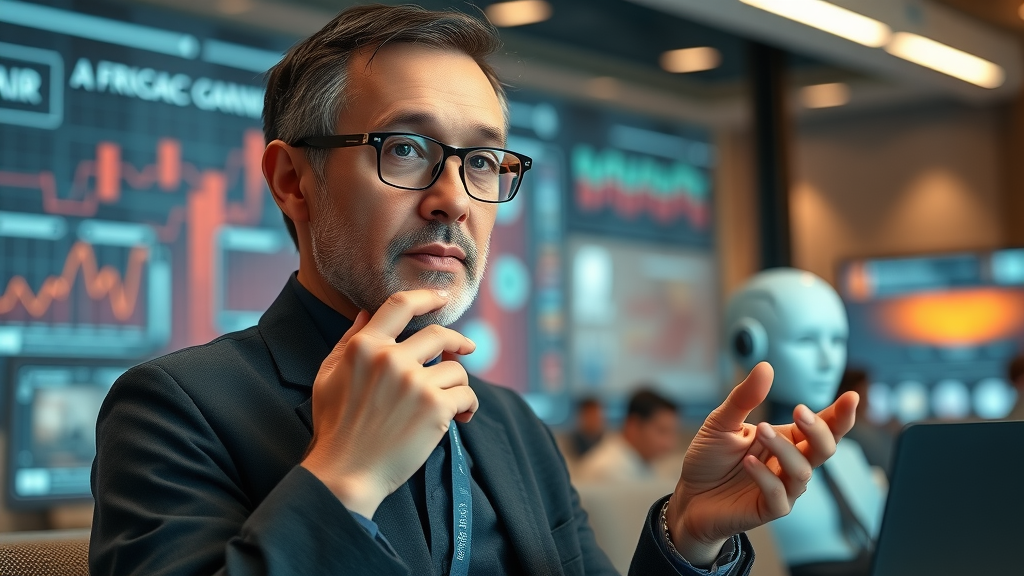
Answer: Delineating the Core Differences and Applications
The key difference lies in data processing and task complexity. Machine learning relies on explicit instructions and structured data, whereas deep learning uses neural networks to interpret unstructured data autonomously, rendering it ideal for more complex, high-dimension data tasks.
Is ChatGPT machine learning or deep learning?
Answer: Assessing ChatGPT's Learning Framework
ChatGPT utilizes deep learning algorithms. Its framework is built upon extensive neural networks, allowing it to understand and generate human-like dialogue effectively. This illustrates deep learning's prowess in natural language processing tasks.
Should I take machine learning or deep learning?
Answer: Guiding Factors for Choosing Between Machine Learning and Deep Learning
Choosing between machine learning and deep learning depends on your goals. If working with smaller data sets and needing quicker deployment, machine learning is suitable. For tasks requiring extensive data analysis and higher precision, deep learning is the better ally.
Is CNN deep learning or machine learning?
Answer: Exploring CNN's Position in the Learning Spectrum
Convolutional Neural Networks (CNNs) are considered a part of deep learning. They are specialized in processing data with a grid-like topology, making them ideal for image and video recognition tasks due to their ability to capture spatial hierarchies in data.
The Impact of Supervised Learning in AI Developments
Supervised learning bridges the gap between machine learning and deep learning, offering methods that train systems using input-output pairs to improve accuracy and efficiency in data processing.
Supervised Learning: Bridging Machine and Deep Learning
Employing supervised learning techniques allows both machine learning and deep learning models to evolve through labeled data. These models enhance their decision-making capabilities, fostering advancements in AI solutions across multiple sectors.
Integrating Supervised Learning in AI Solutions
Supervised learning forms an integral part of AI solutions, ensuring models receive accurate data mapping for effective decision-making. Its structured approach enables enhanced performance in applications like voice recognition, autonomous vehicles, and predictive analytics.
What You'll Learn: Navigating the Complex Landscape of Learning Algorithms
Essential Insights into Machine Learning v Deep Learning
Through this exploration, we've highlighted the foundational aspects of machine learning and the advanced nuances of deep learning, uncovering their distinct uses and intertwined evolution.
Tables: Comparative Analysis of Learning Methods
The table below illustrates key differences, examining learning models, data requirements, and computational needs for both machine learning and deep learning:
Aspect | Machine Learning | Deep Learning |
---|---|---|
Data Processing | Structured Data | Unstructured Data |
Human Intervention | Required | Minimal |
Computational Power | Low to Moderate | High |
Quotes: Expert Opinions on AI Innovations
"Deep learning transcends the capabilities of machine learning by autonomously unraveling complex data patterns, heralding a new era in AI sophistication." - Dr. A.I. Pioneer
Lists: Key Takeaways from Machine Learning v Deep Learning
- Machine Learning requires human input for feature mapping, suitable for smaller datasets.
- Deep Learning leverages neural networks to handle complex, high-volume datasets with precision.
- Both technologies play a pivotal role in the continuous advancement of AI solutions.
FAQs: Addressing Common Queries on Learning Technologies
The complexities of machine learning and deep learning spark curiosities about their applications and implications. By addressing these FAQs, one gains a clearer understanding of how these technologies revolutionize modern industries.
Write A Comment